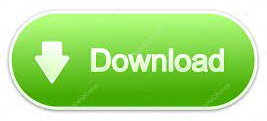
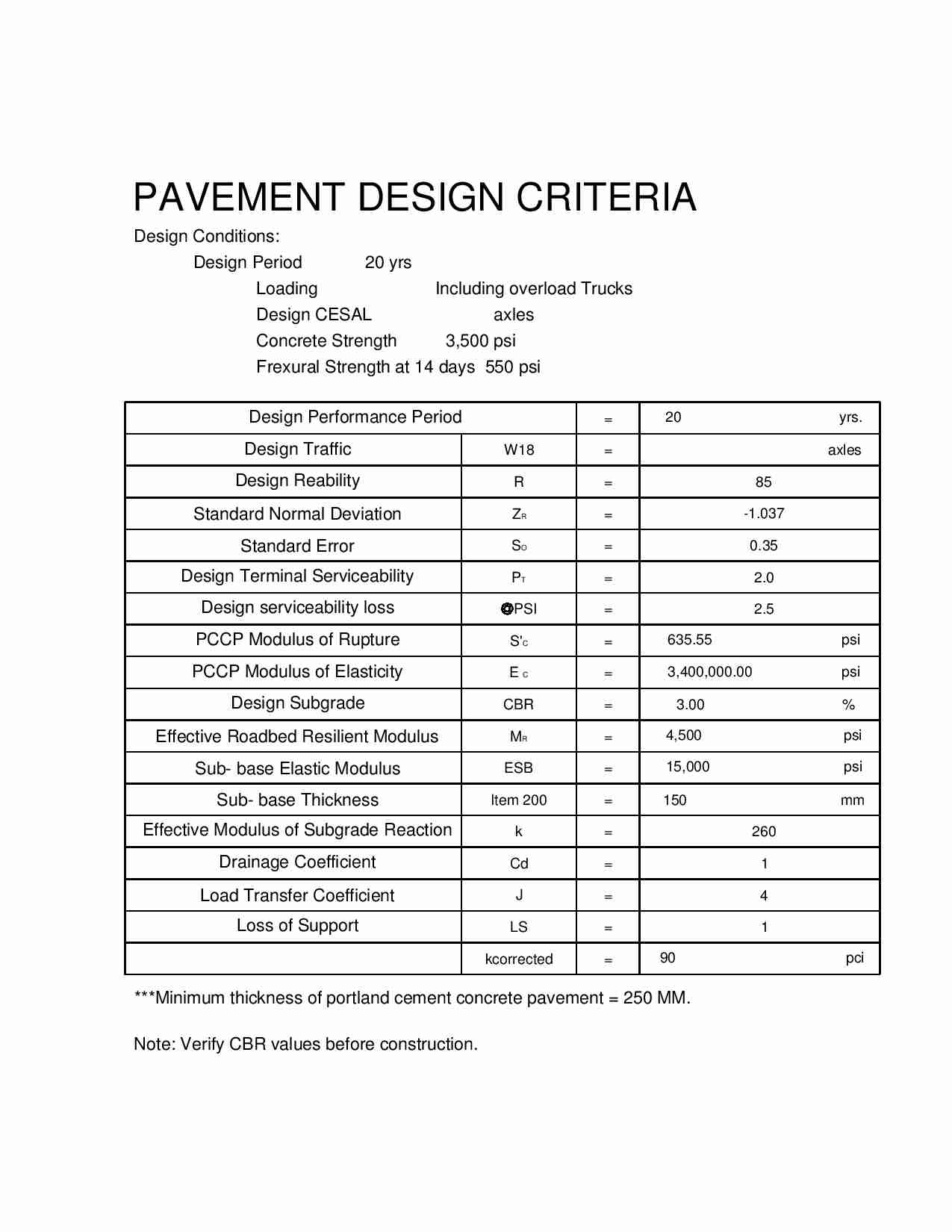
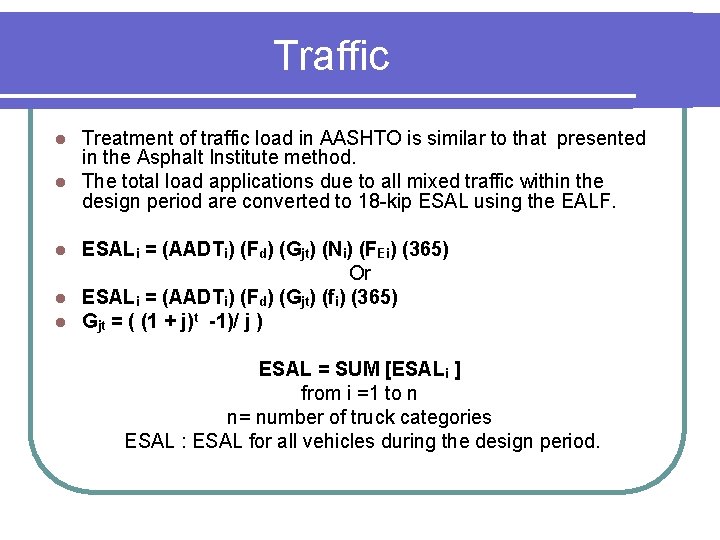
When considering the overall performance of HIS pavements, we find that most of the pavements have lasted the expected 20 years while carrying traffic volumes far in excess of those predicted at the time of design. The results of the AASHO road test have served as the basis for nearly all subsequent pavement designs used in the original construction of the interstate highway system (IHS) after 1961. The AASHO road test was probably the most significant piece of pavement research performed in the 20 th century. (SHRP-P-394, ISBN 3-1, Product No.: 5000).įunding: The author has no support or funding to report.Ĭompeting interests: The author has declared that no competing interests exist. All relevant data are within the paper and Reference 4: “Evaluation of the AASHTO Design Equations and Recommended Improvements,” Strategic Highway Research Program, National Research Council, Washington, DC, 1994. This is an open-access article distributed under the terms of the Creative Commons Attribution License, which permits unrestricted use, distribution, and reproduction in any medium, provided the original author and source are credited.ĭata Availability: The author confirms that, for approved reasons, some access restrictions apply to the data underlying the findings. Received: AugAccepted: OctoPublished: November 14, 2014Ĭopyright: © 2014 Mesut Tiğdemir. PLoS ONE 9(11):Įditor: Wen-Bo Du, Beihang University, China It is concluded that the neural network may be an appropriate tool for the development of databased-nonparametric models of pavement performance.Ĭitation: Tiğdemir M (2014) Re-Evaluation of the AASHTO-Flexible Pavement Design Equation with Neural Network Modeling. Here we aimed to demonstrate that the proposed neural network model can more accurately represent the loads values data, compared against the performance of the AASHTO formula. The existing AASHTO flexible pavement design equation does not currently predict the pavement performance of the strategic highway research program (Long Term Pavement Performance studies) test sections very accurately, and typically over-estimates the number of equivalent single axle loads needed to cause a measured loss of the present serviceability index.
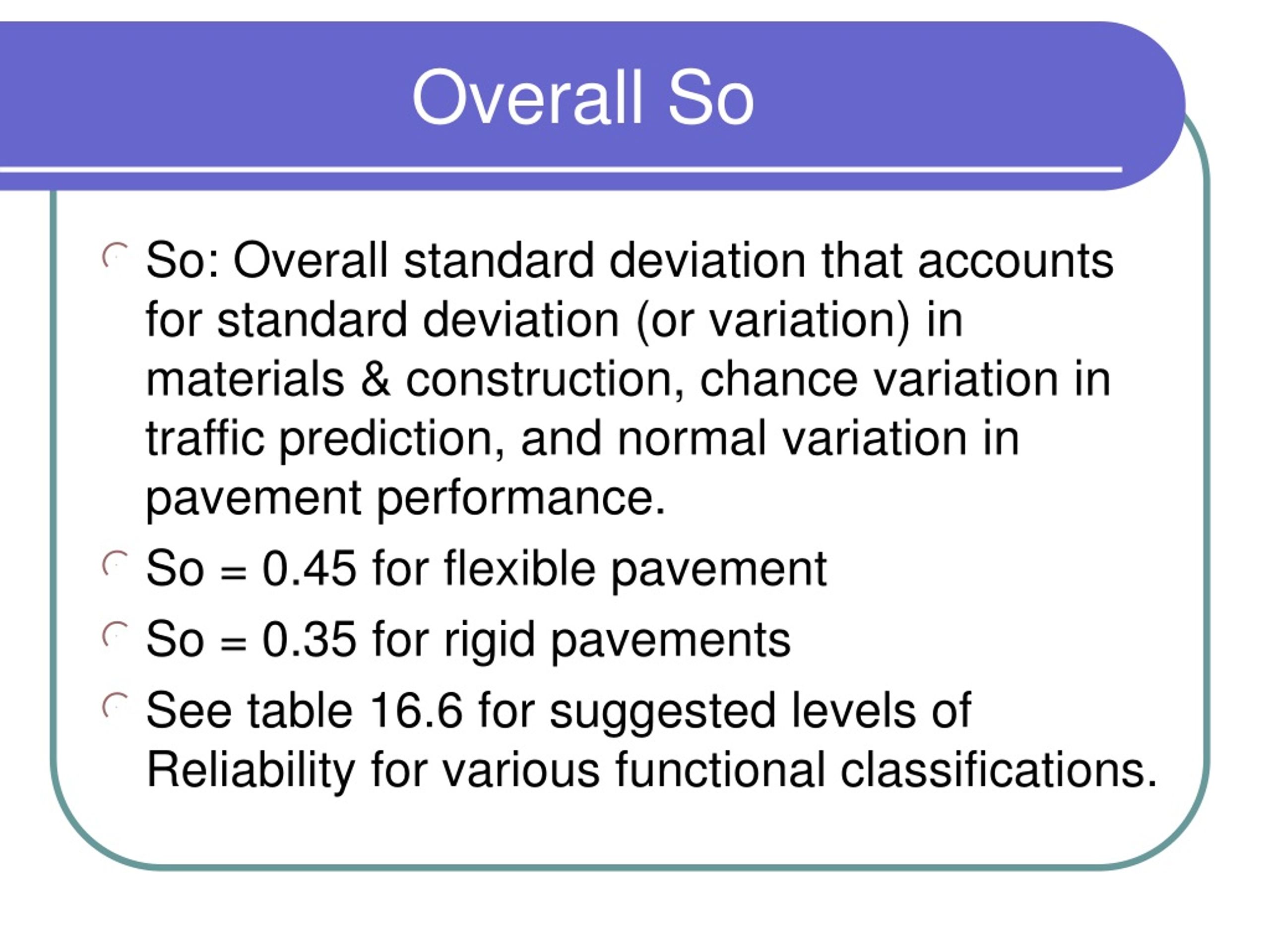
The artificial neural network method is used for this purpose. Thus, those design traffic values that might result in deterioration can be better calculated using the neural networks model than with the AASHTO design equation. More importantly, we find that the neural network model gives the coefficients to be able to obtain the actual load values using the AASHTO design values. Here we establish that equivalent single-axle loads values can be estimated using artificial neural networks without the complex design equality of American Association of State Highway and Transportation Officials (AASHTO).
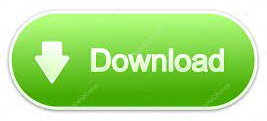